Gaping Data Gap: Addressing Gender Disparities in AI Healthcare and Medicine
Artificial intelligence (AI) has revolutionized healthcare and medicine, offering the potential to improve diagnosis, treatment, and patient outcomes. However, a significant gender disparity exists in the data used to train and develop AI algorithms in these fields. This data gap poses a substantial challenge, as it perpetuates biases and limits the effectiveness of AI technologies for women. In this blog, we will explore the reasons behind the data gap between men and women in AI healthcare and medicine and discuss the importance of addressing this issue for better gender equity and improved healthcare outcomes.
The data gap in AI healthcare and medicine stems from several factors. Historically, medical research has predominantly focused on male subjects, leading to a lack of gender-specific data for women. This bias in research is reflected in the datasets used to train AI algorithms, resulting in models that are less accurate and less effective in diagnosing and treating female patients.
Additionally, women's health issues, such as reproductive health and hormonal variations, are often more complex and less understood than men's health. Consequently, the data available on women's health conditions and responses to treatments is limited, further contributing to the data gap.
The Consequences of the Data Gap:
The data gap in AI healthcare and medicine has significant implications for women's health. Biased algorithms can lead to misdiagnosis, ineffective treatment plans, and delayed interventions for women. For example, studies have shown that AI algorithms used in breast cancer screening tend to be less accurate for women with dense breast tissue, resulting in missed diagnoses.
Moreover, the lack of gender-specific data hinders the development of personalized medicine for women. Treatment plans often rely on data derived from male patients, potentially leading to ineffective or inappropriate interventions for women. This gap perpetuates healthcare disparities and undermines efforts to deliver patient-centered care.
Addressing the Data Gap:
Bridging the data gap between men and women in AI healthcare and medicine is essential for achieving gender equity and improving healthcare outcomes for all. Here are some strategies to address this issue:
1. Diverse and Inclusive Data Collection: Researchers and healthcare institutions should prioritize collecting diverse and representative data that includes a sufficient number of women across different age groups, ethnicities, and health conditions. This effort will ensure that AI algorithms are trained on comprehensive datasets that accurately reflect the diverse population they aim to serve.
2. Gender-Specific Research: Increased funding and support for gender-specific research are necessary to advance our understanding of women's health. This includes studying conditions that predominantly affect women, exploring the impact of hormonal variations, and investigating sex-specific responses to treatments.
3. Collaboration and Partnerships: Collaboration between healthcare professionals, AI researchers, and policymakers is crucial to address the data gap. By working together, stakeholders can develop guidelines and standards for gender equity in AI healthcare and medicine, ensuring that gender biases are identified, addressed, and eliminated.
4. Ethical Considerations: AI algorithms should be designed with ethical considerations in mind, focusing on transparency, accountability, and fairness. Regular audits and evaluations of algorithms should be conducted to identify and correct any biases that may arise.
5. Education and Awareness: Raising awareness about the data gap and its consequences is essential. By educating healthcare providers, researchers, and the general public about the importance of gender equity in AI healthcare and medicine, we can foster a collective commitment to addressing this issue.
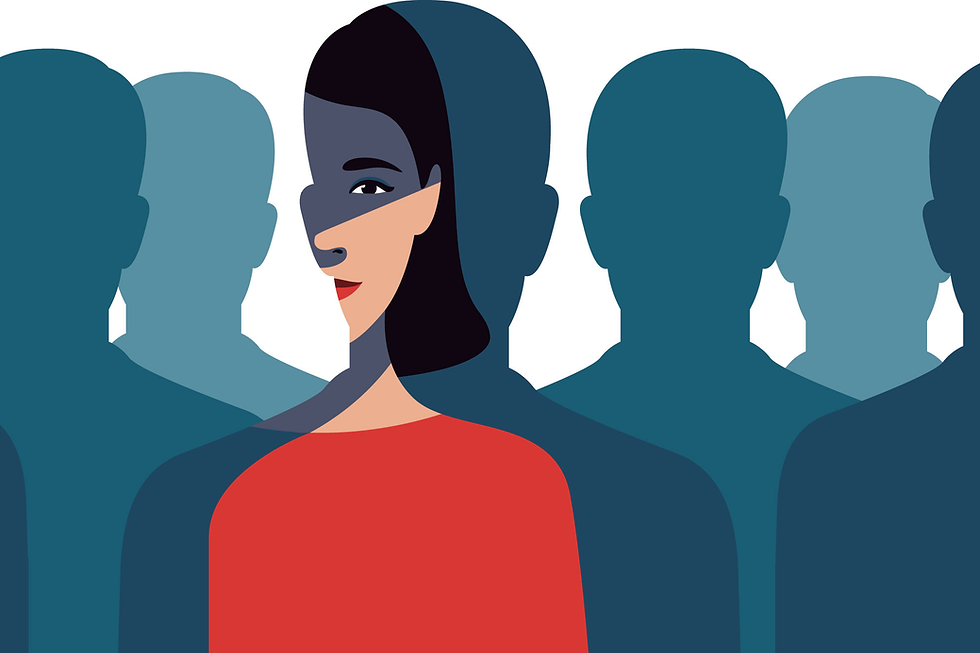
Closing the data gap between men and women in AI healthcare and medicine is a crucial step towards achieving gender equity and improving healthcare outcomes for all. By recognizing the biases inherent in the data used to train AI algorithms, and implementing strategies to collect diverse and inclusive data, we can develop AI technologies that are more accurate, effective, and equitable. By embracing collaboration, ethical considerations, and increased research on women's health, we can bridge the data gap and pave the way for a future where AI technologies benefit women as much as men, ultimately leading to better healthcare for all.
Anaya this is really good information.. how are you planning to reach the millions of women who need this info but cannot read the English language.. Nayan Parekh